Information Technology
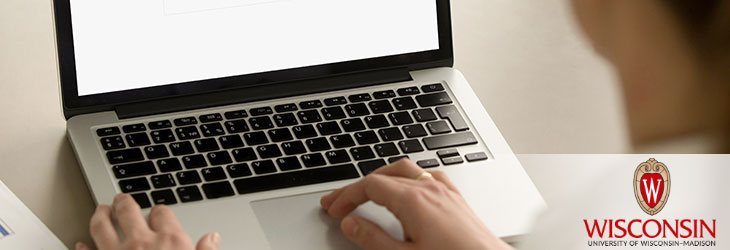
An Improved Method for Analyzing Wireless Broadcasts
WARF: P09135US
Inventors: Akbar Sayeed, Robert Nowak, Waheed Bajwa, Jarvis Haupt
The Wisconsin Alumni Research Foundation (WARF) is seeking commercial partners interested in developing a more efficient method for recovering channel state information.
Overview
In wireless telecommunications, signals are transmitted and received over transmission channels within a limited range of frequencies, typically between 0.7 GHz to 6 GHz for domestic applications. Due to the limited amount of transmission space, it is increasingly important to perfect techniques that estimate channel state information (CSI) at the transmitter and/or receiver to maximize wireless performance over the multipath channels. In modern communication systems training-based channel learning techniques are commonly used to retrieve the CSI at the receiver, which can improve communication efficiency, particularly in terms of power and spectral efficiency.
Training-based methods probe the receiver channel with a multiplexed signal, consisting of known signal waveforms and information bearing signals, and process the corresponding channel output to learn the CSI. Multipath channels tend to exhibit an approximately sparse multipath structure at high signal space dimension (time-bandwidth-antenna product), where the CSI has significantly fewer dominant parameters. Due to the inherently low-dimensional nature of sparse multipath channels, conventional training-based methods are not appropriate since they are based on exhaustive probing coupled with a complex estimation approach comprising linear least squares or non-linear parametric estimators.
To accurately learn the channel response at the receiver and increase communication efficiency at high signal space dimensions, a new method is needed that will provide a fast response time, more efficient estimation strategies and training waveforms that are tailored for sparse multipath channel applications.
Training-based methods probe the receiver channel with a multiplexed signal, consisting of known signal waveforms and information bearing signals, and process the corresponding channel output to learn the CSI. Multipath channels tend to exhibit an approximately sparse multipath structure at high signal space dimension (time-bandwidth-antenna product), where the CSI has significantly fewer dominant parameters. Due to the inherently low-dimensional nature of sparse multipath channels, conventional training-based methods are not appropriate since they are based on exhaustive probing coupled with a complex estimation approach comprising linear least squares or non-linear parametric estimators.
To accurately learn the channel response at the receiver and increase communication efficiency at high signal space dimensions, a new method is needed that will provide a fast response time, more efficient estimation strategies and training waveforms that are tailored for sparse multipath channel applications.
The Invention
UW-Madison researchers have developed a more efficient method for recovering CSI. This method uses a non-linear reconstruction algorithm that learns multipath channels in time, frequency and space. By tailoring training signals and estimation strategies to the anticipated characteristics of the underlying channel, the researchers were able to yield better estimates than conventional procedures.
This approach improves the accuracy of learning the receiver channel response by focusing on two critical aspects of training-based channel learning methods, sensing and estimation. Sensing relates to the design and placement of training signals that are used to probe the channel. This method defines the most suitable transmitter training signals for exploiting multipath sparsity in angle, delay and/or Doppler. Estimation refers to the signal analysis process implemented at the receiver to recover the channel response. Researchers implemented non-linear reconstruction algorithms, based on convex/linear programming, that come within a logarithmic factor of the performance of an ideal channel estimator. This new approach clearly reveals how the relationship between the training signals and the accuracy of this algorithm efficiently estimates the CSI, resulting in faster channel responses at the receiver.
This approach improves the accuracy of learning the receiver channel response by focusing on two critical aspects of training-based channel learning methods, sensing and estimation. Sensing relates to the design and placement of training signals that are used to probe the channel. This method defines the most suitable transmitter training signals for exploiting multipath sparsity in angle, delay and/or Doppler. Estimation refers to the signal analysis process implemented at the receiver to recover the channel response. Researchers implemented non-linear reconstruction algorithms, based on convex/linear programming, that come within a logarithmic factor of the performance of an ideal channel estimator. This new approach clearly reveals how the relationship between the training signals and the accuracy of this algorithm efficiently estimates the CSI, resulting in faster channel responses at the receiver.
Applications
- Can be incorporated into wireless communication systems, including radar and underwater communication systems
- Analyzes “white space,” or unused radio frequencies, to provide improved signal transmission efficiency and bandwidth, while reducing destructive interference from adjacent signals
Key Benefits
- Improves accuracy and efficiency of wireless communication
- Reduces power/energy and bandwidth expenditure by more than 50 percent
- Provides lower latency in data transmission due to the higher fraction of bandwidth available for communication
- Provides improved robustness to noise and signal errors
Stage of Development
The development of this technology was supported by the WARF Accelerator Program. The Accelerator Program selects WARF’s most commercially promising technologies and provides expert assistance and funding to enable achievement of commercially significant milestones. WARF believes that these technologies are especially attractive opportunities for licensing.
Additional Information
For More Information About the Inventors
Related Technologies
For current licensing status, please contact Jeanine Burmania at [javascript protected email address] or 608-960-9846