Medical Imaging
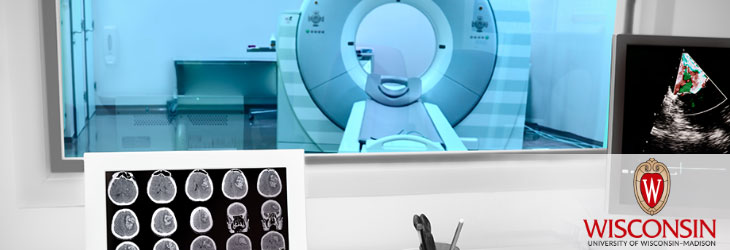
Method for Data-Consistency Preparation and Improved Image Reconstruction
WARF: P170192US01
Inventors: Guang-Hong Chen
The Wisconsin Alumni Research Foundation (WARF) is seeking commercial partners interested in developing a new method for processing CT and other medical imaging data to promote consistency and reconstruct images with controlled artifacts.
The method is used to create subsets of data with a high level of data consistency to guide image reconstruction. The data subsets can then be reconstructed into images with substantially reduced artifacts.
Overview
Routinely, data acquired with medical imaging systems are not consistent with a single true image of the subject. Data inconsistencies arise from various causes (beam-hardening effects, subject motion, etc.) and manifest as artifacts in the reconstructed images.
Although a qualitative correlation between data inconsistency and the resultant artifacts is known, there are no widely accepted, systematic ways to quantify the relationship between artifacts and data inconsistency levels. Nor is there a method to develop image reconstruction algorithms that incorporate data consistency evaluation and classification into the reconstruction process to sufficiently mitigate artifacts.
Needed is a new method capable of characterizing intrinsic data consistency levels from one datum to another, or from one view of data to another, and to incorporate the new data consistency classification into image reconstruction. This could yield images that are free from or otherwise not corrupted by artifacts, despite inconsistent and/or undersampled data.
Although a qualitative correlation between data inconsistency and the resultant artifacts is known, there are no widely accepted, systematic ways to quantify the relationship between artifacts and data inconsistency levels. Nor is there a method to develop image reconstruction algorithms that incorporate data consistency evaluation and classification into the reconstruction process to sufficiently mitigate artifacts.
Needed is a new method capable of characterizing intrinsic data consistency levels from one datum to another, or from one view of data to another, and to incorporate the new data consistency classification into image reconstruction. This could yield images that are free from or otherwise not corrupted by artifacts, despite inconsistent and/or undersampled data.
The Invention
Recognizing the link between data consistency and artifact mitigation, UW–Madison professor Guang-Hong Chen has developed an improved processing method that is applicable across modalities, including CT imaging, PET, SPECT and MRI.
The new method provides a practical means to define a data inconsistency metric (DIM) that can be used to locally characterize the inconsistency level of each acquired datum or a view of acquired data. The DIM can be used in a data classification technique to select an optimal data set with a minimal data inconsistency level to reconstruct images with minimal artifact contamination.
The acquired datasets can be classified into one or more subsets based upon the value of DIM. After data classification, a reconstruction technique, such as the SMART-RECON algorithm pioneered by Prof. Chen, can be used to reconstruct these sub-images. Each sub-image is consistent with the subset of the projection view angles for a given range of DIM values. The result is substantially improved images.
The new method provides a practical means to define a data inconsistency metric (DIM) that can be used to locally characterize the inconsistency level of each acquired datum or a view of acquired data. The DIM can be used in a data classification technique to select an optimal data set with a minimal data inconsistency level to reconstruct images with minimal artifact contamination.
The acquired datasets can be classified into one or more subsets based upon the value of DIM. After data classification, a reconstruction technique, such as the SMART-RECON algorithm pioneered by Prof. Chen, can be used to reconstruct these sub-images. Each sub-image is consistent with the subset of the projection view angles for a given range of DIM values. The result is substantially improved images.
Applications
- Medical image reconstruction software
- Universal modality (tomographic and non-tomographic systems)
- Especially useful for specific anatomical imaging where motions are prevalent (e.g., cardiac, respiratory or bolus injection)
Key Benefits
- Potential to provide best reconstruction possible for any given data set
- Does not depend on a specific data acquisition geometry/physics model
Stage of Development
Actual patient data sets have been reconstructed for a variety of CT data sets: metal implants, motion artifacts and beam hardening.
Additional Information
For More Information About the Inventors
Related Technologies
For current licensing status, please contact Jeanine Burmania at [javascript protected email address] or 608-960-9846