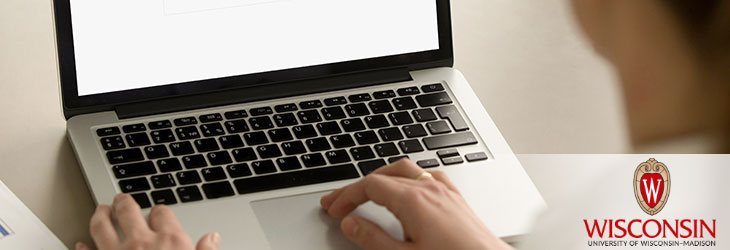
UW-Madison researchers have created new learning-based compressive spatio-temporal histograms for high-resolution single-photon 3d cameras. They created a family of compressive representations for histogram images that can be computed in an online fashion with limited memory and compute requirements. They are based on the linear spatiotemporal projection of each photon timestamp, which can be expressed as a simple matrix operation. Instead of storing the full timestamp spatial (pixel location) and temporal information on a per-pixel histogram, a compressive encoding maps its spatio-temporal information onto a compressive histogram. To exploit local spatiotemporal correlations, a single compressive histogram is built for a local 3D histogram block. Therefore, instead of building and storing the full 3D histogram image in-sensor, multiple compressive histograms are built and transferred off-sensor for processing, effectively reducing the required in-sensor memory and data rates. For different datasets, a neural network model can use this SPAD timestamp histogram data and this CSPH framework can be integrated within the model and jointly optimize the CSPH representation with the task-specific model.