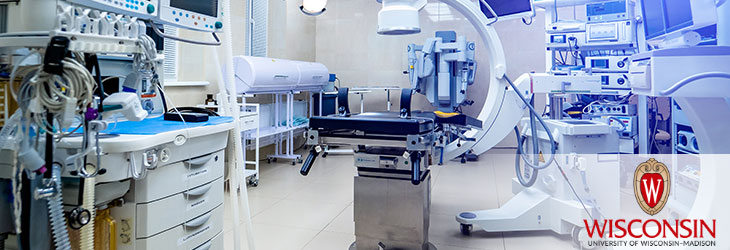
Recent developments for measuring gene expressions in tissues promise to improve our understanding of genetic disorders and idiopathic conditions. Of particular interest are spatial transcriptomics platforms which allow monitoring of gene expression in different locations within the tissue at a near cellular level. Such tools can produce large amounts of data covering many different gene expressions at thousands of tissue points. Despite this improved access to important gene expression data, spatial transcriptomics has had limited success in refining diagnoses or identifying appropriate therapies.
UW-Madison researchers have developed a software tool that greatly improves the ability of a clinician to make use of the large amounts of data produced by spatial transcriptomics. The system augments a transcriptomics platform showing gene expression measurements with a registered image of a stained tissue providing improved insight into the spatial context of the gene expression, for example, according to cell types or other structures within a tissue. It is also capable of evaluating and quantify similarities and differences in gene expressions from different tissue samples.
Importantly, spatial transcriptomics data can be augmented with cell type information, such as cell function, and input into a database that may be used to identify other tissue samples that are highly similar and for which diagnosis or treatment options are known. By exploiting the powerful pattern matching capabilities of machine learning or other computational approaches, the wealth of data obtained from spatial transcriptomics may be harnessed to enhance diagnostic or treatment information, independent of a complete understanding of the underlying cellular mechanisms. Using this information, clinicians may match subsequent and different tissue samples based on clinical information and histologic patterning in a comprehensive way to obtain insights into diagnosis and treatment from existing
and trained data.